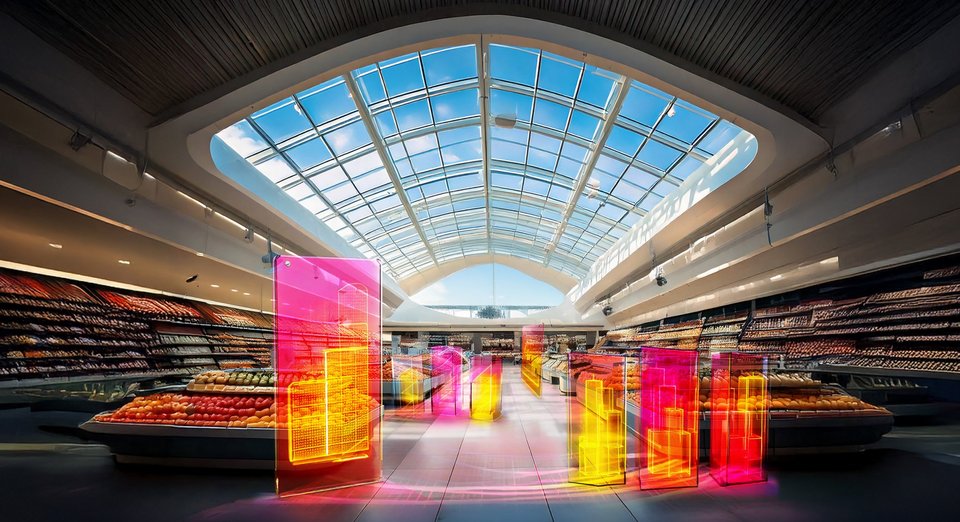
Artificial intelligence (AI) is revolutionising data analysis in companies, especially in the retail sector. By using AI technologies, companies can make informed decisions, increase customer satisfaction or improve the efficiency of stock management. In order to better understand data-driven decisions with the help of AI in our company, we spoke to the Head of Analytics, Dr Lorenz Determann.
We recognised the benefits of AI early on and decided to integrate it as a complementary value driver in as many areas of the company as possible. We launched the first applications in the areas of personalisation and product range optimisation as early as 2018, and with the Analytics Transformation 2021, AI was also strategically and organisationally anchored in REWE Group.
The use of AI in data analysis enables us to recognise complex patterns. In practice, this can be seen in the stores in shelf maintenance or space optimisation. Here, analytical AI has proven to be an invaluable tool. Another example is the search for matching items to customer needs such as "reduced-sugar items" or "Asian cooking".
By using AI, we can significantly improve our customers' shopping experience in our food retailers. With the help of AI, we analyse sales data and customer behaviour anonymously to determine exactly which products need to be available at the right place at the right time. Overall, this not only leads to greater customer satisfaction, but also to less food spoilage, more efficient stock management and reduced costs.
Lorenz Determann
one: How long have we been using AI in data analysis and how has its use developed over time?
Lorenz Determann : We started using data warehousing at REWE Group back in 2002. Even back then, the aim was to improve our decisions by processing large amounts of data. AI has multiplied both the possible applications and the benefits of data analyses in all areas of the company. Specifically, there are three main drivers that have changed since 2002: the storage and analysis of data has become almost infinitely scalable and significantly cheaper thanks to new cloud technologies. In addition, AI processes are no longer part of expensive software packages, but can be downloaded free of charge from the internet. What's more, the number of well-trained young talents has increased significantly, allowing us to expand our workforce in addition to technical scaling.
one: How exactly does AI help us to optimise the shopping experience in our food retailers?
Lorenz Determann : A good example is the HOLMES project, in which we specifically look at transaction data in the stores and analyse it with the help of AI. This allows us to recognise certain patterns, which we then use to explain anomalies in sales. Let's assume someone buys a spice mix for Mac&Cheese, milk and grated cheese, but not macaroni. The AI then recognises the missing product based on a previously trained model of item relationships and thus helps to identify gaps on the shelf or items that are not in the right place more quickly.
The prerequisite for this was that we first "fed" the AI with billions of lines of till receipts so that it could learn patterns. The AI is now continuing to refine these and is becoming better and better at recognising anomalies. This is also supported by the store employees, who provide digital feedback on whether the AI has recognised anomalies correctly without any additional effort (this is how HOLMES finds missing items - one). Together with the AI, we can significantly improve the availability of articles in the stores.
one: What challenges did or do you face when integrating AI into our existing systems?
Lorenz Determann : One challenge is good data. Modern AI processes require up-to-date, detailed and quality-assured data in order to make precise predictions and recognise correlations. In the simpler methods of the past, a lot of calculations were based on averages, so an outlier in a market didn't carry much weight - with market-specific recommendations of articles, a different level of accuracy is required today. Another challenge is the integration of analytical suggestions into the operational systems without system breaks and duplicate entries. One example of how we have successfully mastered this challenge is a technical solution that transfers price suggestions directly into the input masks for operational price maintenance.
one: What future developments and innovations do you see in the field of AI-supported data analysis?
Lorenz Determann: The better AI gets, the more use cases we will find. And new options are emerging practically every day. Since AI is able to solve problems independently based on available information - known as reasoning in AI parlance - completely new possibilities are opening up. AI agents that independently collect, analyse and communicate information within a defined framework, for example via interfaces or databases, are the next exciting step in development right now. But the question: "How do you ensure that reliable and up-to-date information is displayed in ChatGPT and the like?" is also currently an open issue.